Qualcomm Innovation Fellowship Europe in 2024 Rewards Excellent Research in the Field of AI and Cybersecurity
Qualcomm Technologies announced the winners of the 2024 Qualcomm Innovation Fellowship (QIF) Europe, rewarding PhD students excelling in AI and cybersecurity. Each winner will receive $40,000 and mentorship from Qualcomm's research team. This year's winners include students from EPFL, University of Cambridge, and ETH Zurich. Notable projects involve advancements in virtual reality, confidential computing, 3D RNA design, controlled text generation, and deep learning curvature matrices. The program saw nearly 50% more submissions, highlighting the growing significance of machine learning and cybersecurity.
- Qualcomm awarded $40,000 and mentorship to each winner, showcasing investment in future tech talent.
- There was a nearly 50% increase in submissions, indicating robust interest and innovation in AI and cybersecurity.
- Selected winning projects cover breakthrough ideas in virtual reality, confidential computing, 3D RNA design, controlled text generation, and deep learning.
- None.
NORTHAMPTON, MA / ACCESSWIRE / July 9, 2024 / Qualcomm:
Each Winner Receives Mentorship and
Qualcomm Technologies, Inc., announced the winners of the Qualcomm Innovation Fellowship (QIF) Europe program, now in its 15th year: Dongqing Wang (EPFL), Neelu S. Kalani (EPFL), Chaitanya K. Joshi (University of Cambridge), Runa Eschenhagen (University of Cambridge), and Afra Amini (ETH Zürich)
QIF is an annual program that focuses on recognizing, rewarding, and mentoring the most innovative engineering PhD students across Europe, India, and the United States. The European program rewards excellent young researchers in the fields of artificial intelligence and cybersecurity with individual prizes of
"This year we received almost
The seventeen finalists were PhD candidates from ETH Zurich, Imperial College London, University of Edinburgh, Tübingen University, University of Cambridge, University of Oxford, CISPA Helmholtz Center for Information Security, TU Delft, and Czech Technical University.
After careful review, the following five winners were selected for their outstanding proposals:
"Towards visually plausible and controllable 360° Virtual Reality" - Dongqing Wang
Creating visually plausible and controllable virtual reality (VR) of the real world is key to enabling quality immersive experiences in extended reality (XR) applications. NeuralRadiance Fields (NeRF) and their variants can model 360-degree real-world scenes for photorealistic novel view synthesis with low memory storage. Consequently, they have the potential to become widely accessible 3D world representations. However, the implicit nature of their underlying representation makes it challenging to directly edit a 3D NeRF scene. For controllability, we propose a 3-component strategy to enable an editing system on NeRF. The outcome of this proposal will aim to enable a visually plausible and controllable 360-degree virtual reality to enhance virtual world interaction.
"FlashPoint: Secure Dynamic Root of Trust " - Neelu S. Kalani
The confidential computing field has seen significant advancements over the past decade. Even as confidential computing evolves, minimising the trusted compute base (TCB) remains crucial. So far, little focus has been put towards removing platform-specific firmware, that executes with the highest privileges alongside security monitors that provide confidential computing guarantees, from the TCB. In the meantime, numerous vulnerabilities in the large and buggy platform-specific firmware have been exploited (e.g. to leak the platform's secret keys) to compromise the entire system's security. We propose FlashPoint, a dynamic root of trust solution for RISC platforms. It comprises of ISA extensions to enable secure transitions between trusted and untrusted code executing in the highest privilege mode, without introducing a new privilege mode.
"Geometric Generative Models for 3D RNA Design" - Chaitanya K. Joshi
This proposal aims to develop the first deep learning framework for 3D RNA design. I will outline an execution plan for an RNA-centric 3D generative model that builds upon best practices that have revolutionized protein design. I will discuss why AlphaFold is not enough, how to address RNA-specific modelling challenges, and why incorporating inductive biases that drive RNA structure are critical to develop bespoke generative models for RNA design.
"An Adventure Towards Effective Controlled Text Generation" - Afra Amini
Imagine a scenario in which you are using the language model of your choice to generate a fictional story. You ask the language model to generate a story about a TikZ unicorn coming to life, and the model outputs a story. While being fascinated by this amazing technology, you realize that the story is too short for your purpose, the language that is used is very formal and not suited for your target audience, and the sentence structures are too complex. How can you systematically control these aspects in the generated story? Which knobs of the model do you have to tweak to ensure the generations satisfy the desired constraints? In this work, we explore recent advances in two research directions for systematically controlling text generation. We also demonstrate how these two approaches can be unified as different methods for approximating the same objective.
"Towards Understanding Curvature Matrices in Deep Learning" - Runa Eschenhagen
Many algorithms trying to address shortcomings of deep learning rely on approximations of the Hessian of the loss with regards to the neural network's parameters or other related quantities, so-called curvature matrices. This includes second-order optimization methods for improved training efficiency, influence functions for data attribution, methods for pruning and compression, predictive uncertainty quantification, and more. However, the effect of the curvature approximation on downstream task performance is not well understood. My proposal aims to improve our understanding of commonly used curvature approximations, specifically variations of K-FAC. This has the potential to directly impact all applications that rely on these approximations, inform the design of new approximations, provide insights into training dynamics, and lay the foundation for new theoretical explanations.
About Qualcomm
Qualcomm relentlessly innovates to deliver intelligent computing everywhere, helping the world tackle some of its most important challenges. Our proven solutions drive transformation across major industries, and our Snapdragon® branded platforms power extraordinary consumer experiences. Building on our nearly 40-year leadership in setting industry standards and creating era-defining technology breakthroughs, we deliver leading edge AI, high-performance, low-power computing, and unrivaled connectivity. Together with our ecosystem partners, we enable next-generation digital transformation to enrich lives, improve businesses, and advance societies. At Qualcomm, we are engineering human progress.
Qualcomm Incorporated includes our licensing business, QTL, and the vast majority of our patent portfolio. Qualcomm Technologies, Inc., a subsidiary of Qualcomm Incorporated, operates, along with its subsidiaries, substantially all of our engineering and research and development functions and substantially all of our products and services businesses, including our QCT semiconductor business. Snapdragon and Qualcomm branded products are products of Qualcomm Technologies, Inc. and/or its subsidiaries. Qualcomm patented technologies are licensed by Qualcomm Incorporated.
Contact Information:
Mauricio Lopez-Hodoyan
Investor Relations
1-858-658-4813
ir@qualcomm.com
Clare Conley
Media Relations
1-858-845-5959
corpcomm@qualcomm.com
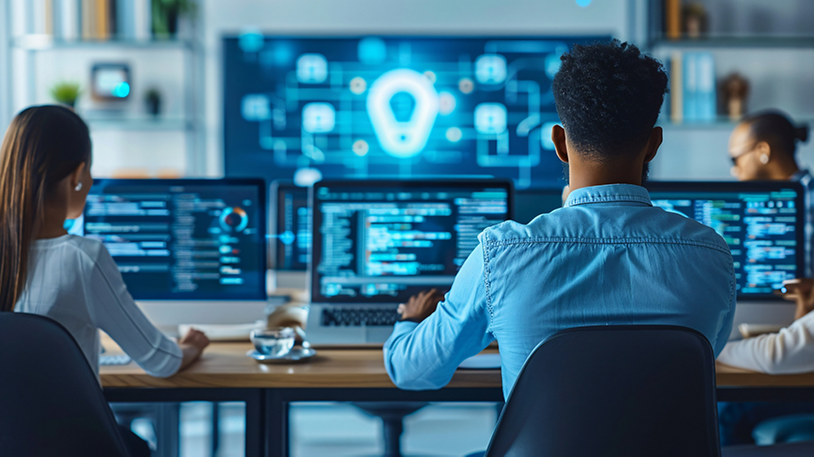
View additional multimedia and more ESG storytelling from Qualcomm on 3blmedia.com.
Contact Info:
Spokesperson: Qualcomm
Website: https://www.3blmedia.com/profiles/qualcomm
Email: info@3blmedia.com
SOURCE: Qualcomm
View the original press release on accesswire.com
FAQ
What is the Qualcomm Innovation Fellowship (QIF) Europe 2024?
Who were the winners of the Qualcomm Innovation Fellowship Europe 2024?
What types of projects won the QIF Europe 2024?
How much funding do QIF Europe 2024 winners receive?